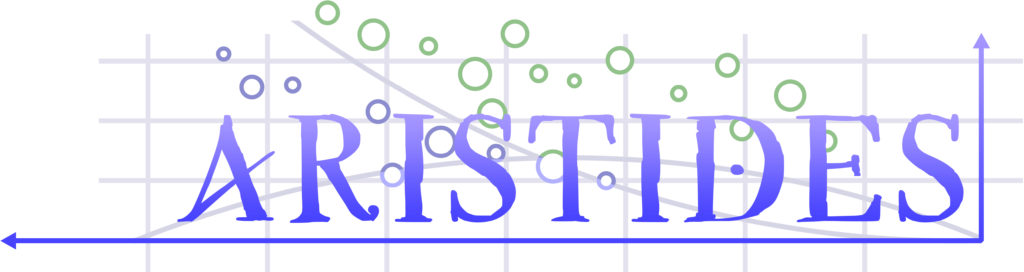
End: 31/08/2022
Funding: National
Status: Completed
Advanced Signal and Information Processing (ASIP)
Acronym: ARISTIDES
Call ID: RTI2018-099722-B-100
Code: RTI2018-099722-B-I00
The ARISTIDES project aims to deepen the theoretical understanding and advance on the performance of data-driven learning and inference algorithms for high-dimensional data processing. A special focus is set on the enhancement of machine learning methods (incl. deep learning) and their application to the re-design of lower-layer functionalities of (beyond) 5G communication systems.
The algorithmic tools investigated include (i) random matrix theory to better model the behavior of kernel-based learning algorithms; (ii) structured sparsity methods to reduce the dimensionality of complex learning problems with a large number of features; (iii) Bayesian inference to enrich learning algorithms with any prior information on the underlying model; and (iv) coded computing strategies to speed up the execution of learning algorithms executed in a distributed manner.
The project will study the applicability of these methods, in combination with other well-known data-driven approaches (e.g., deep learning and reinforcement learning) to the design and optimization of key functionalities in communication systems, with particular emphasis on the PHY layer. Specifically, the ARISTIDES project will (i) investigate and develop data-driven beam/antenna selection and user clustering schemes in mmWave communications for improved performance–complexity trade-off, robustness and scalability; (ii) devise end-to-end learning, autoencoder-inspired techniques for efficient code design for ultrareliable low-latency communication (URLLC); (iii) analyze the feasibility of deep neural network and reinforcement learning-based designs for massive PHY/MAC access schemes; (iv) build a proof-of-concept based on software radio for an end-to-end trained short-packet communication system.
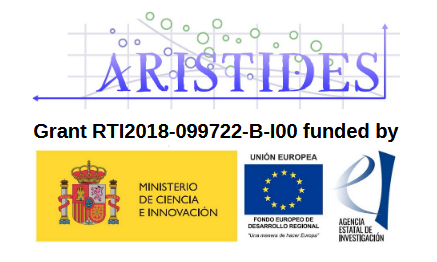
Coordinator